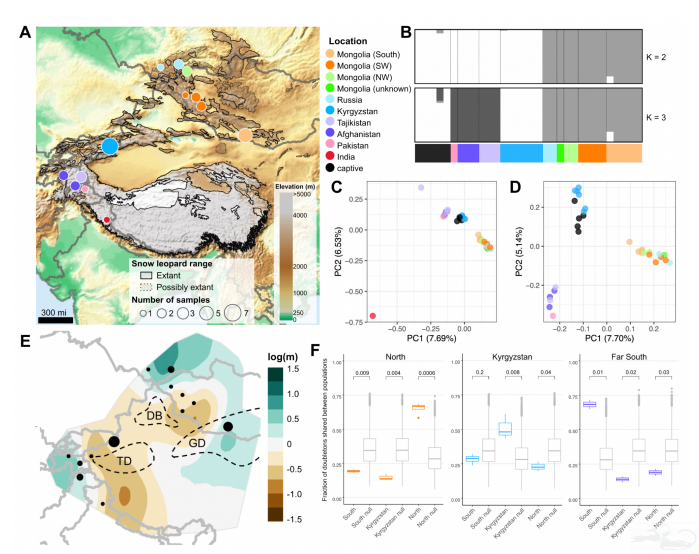
Extreme in Every Way: Exceedingly Low Genetic Diversity in Snow Leopards Due to Persistently Small Population Size
Abstract:
Snow leopards (Panthera uncia) serve as an umbrella species whose conservation
benefits their high-elevation Asian habitat. Their numbers are believed to be in decline due to
numerous Anthropogenic threats; however, their conservation is hindered by numerous
knowledge gaps. They are the least studied genetically of all big cat species and little is known
about their historic population size and range, current population trends, or connectivity across
their range. Here, we use whole genome sequencing data for 41 snow leopards (37 newly
sequenced) to assess population connectivity, historic population size, and current levels of
genetic diversity. Among our samples, we find evidence of a primary genetic divide between the
northern and southern part of the range around the Dzungarian Basin and a secondary divide
south of Kyrgyzstan around the Taklamakan Desert. However, we find evidence of gene flow,
suggesting that barriers between these groups are permeable. Perhaps most noteworthy, we
find that snow leopards have the lowest genetic diversity of any big cat species, likely due to a
persistently small population size throughout their evolutionary history. Without a large
population size or ample standing genetic variation to help buffer them from any forthcoming
Anthropogenic challenges, snow leopard persistence may be more tenuous than currently
appreciated.
Introduction:
Residing in some of the most extreme and remote areas of the world, snow leopards
(Panthera uncia) are rarely encountered and challenging to study, making them one of the most
enigmatic of the large charismatic mammals. Snow leopard habitat consists of mountainous
areas of Asia, spanning 12 countries (Fig. 1A). They are among the largest carnivores in the
high-elevation habitat in which they reside and their persistence relies on healthy mountain
ungulate populations which in turn rely on healthy rangelands1
. Healthy high-elevation
rangeland also benefits pastoral communities and offers immense ecosystem services, acting
as an important source of carbon storage2 and Asia’s water tower. In these regions, snow
leopards serve as an umbrella species whose conservation benefits this globally crucial Asian
mountain ecosystem. However, snow leopard conservation is impeded by the many knowledge
gaps regarding this elusive species1
.
Snow leopards were listed as Endangered by the International Union for Conservation of
Nature (IUCN) for 45 years but were downlisted to Vulnerable in 2017 due to not meeting
specific criteria of population size and percent population decline for Endangered status. This
change of status has been controversial3,4 as snow leopard numbers are presumed to be in
(which was not certified by peer review) is the author/funder. All rights reserved. No reuse allowed without permission.
bioRxiv preprint doi: https://doi.org/10.1101/2023.12.14.571340; this version posted December 15, 2023. The copyright holder for this preprint
3
decline due to habitat loss, decreased availability of primary prey (high-elevation, mountaindwelling ungulates), retaliatory killings for livestock predation5
, and poaching for their skins6
. As
climate change in high mountain Asia is occurring at an even more rapid rate than elsewhere in
the Northern hemisphere7
, it is also likely to become an increasing threat to snow leopards8
.
Currently, the global snow leopard population size is estimated to be anywhere from 4,700-
7,500 individuals and little is known about their historic population size and range9 or their
current population trends10. While many other big cat species experienced historic declines and
are facing a second, contemporary human-driven decline11,12, it remains unclear if the highlyspecialized snow leopard was ever more abundant than is currently estimated. Estimates of
genetic diversity, which are likely to correlate positively with historic population size, are also
limited due to a dearth of genomic data for the species. They are the least studied genetically of
all the big cat species13 with whole-genome sequencing data available for only two wild snow
leopards14 and two captive individuals15 prior to this study. Genetic diversity assessed using
genomic data from one of the previously sequenced wild snow leopards suggests a lack of
diversity14, but additional samples are required to determine if this is a characteristic of the
species across its range.
Additionally, little is known about snow leopard population connectivity across their
range16. A potentially significant gap between northern and southern populations has been
defined in the Dzungarian Basin of northwestern China17,18; however, snow leopards are known
to cross long distances between mountain ranges19,20. To date, most genetic work on snow
leopards has been done using microsatellite markers to estimate population sizes, connectivity,
and diversity at mostly small spatial scales21–26. Using range-wide samples, however, Janečka
et al.27 analyzed 33 microsatellite loci and 683 bp of mitochondrial DNA from fecal samples to
suggest three snow leopard subspecies–one of them occurring north of the Dzungarian Basin
and Gobi Desert and two subspecies south of this divide, separated between the east and west
of the Himalayas-Tibetan Plateau complex. However, this subspecies demarcation and the level
of connectivity across the landscape is controversial28,29. It remains unclear if any landscape
features within the snow leopard range are impermeable or if all snow leopard populations are
connected to some degree through stepping-stone and/or long distance dispersal events.
Here, we generate whole genome sequencing data for 33 wild snow leopards from
multiple locations across their range in addition to four captive snow leopards. We use this
dataset to shed light on two outstanding questions: 1) how connected are snow leopard
populations across their range and 2) what was the historic population size of snow leopards
and how is this reflected in their current levels of genetic diversity. We also compare the genetic
(which was not certified by peer review) is the author/funder. All rights reserved. No reuse allowed without permission.
bioRxiv preprint doi: https://doi.org/10.1101/2023.12.14.571340; this version posted December 15, 2023. The copyright holder for this preprint
4
diversity of snow leopards to that of the other big cats. Among our samples, which do not
include the southeast of the range, we find evidence of a genetic divide between the north and
south, as well as a divide between the Kyrgyz population and populations farther south. Our
data shows evidence of contemporary gene flow, suggesting that barriers between these groups
are permeable. Most notably, we find snow leopards to be the least genetically diverse
contemporary big cat species, likely owed to a persistently small population throughout their
evolutionary history. We believe these results have significant implications for snow leopard
conservation.
Results:
We generated whole genome sequencing data for 37 snow leopards–33 wild born snow
leopards representing seven countries (Mongolia, Russia, Kyrgyzstan, Tajikistan, Afghanistan,
and Pakistan) (Fig. 1A) and four from the American zoo population. We also included all
published whole genome sequencing data for snow leopards in our analysis, which consisted of
data for two additional wild samples, one from Mongolia14 and one from India, and two captive
individuals from the American zoo population15. After removing samples based on sequence
quality and depth we were left with 37 samples–34 from our newly generated data with an
average depth of coverage of 7.3X (minimum of 3X and maximum of 16.8X) and three
previously published samples with an average depth of coverage of 23X (minimum of 12X and
maximum of 28.9X) (Supplementary Table 1).
These data were mapped to the snow leopard reference genome (NCBI accession
PRJNA602938)15 and single nucleotide polymorphisms (SNPs) were called and filtered as
described in the methods, resulting in a final SNP set of 1,591,978. Within this final dataset, we
identified one pair of first-degree relatives and three pairs of second-degree relatives (all from
the wild samples). One representative from each of these four related pairs was removed before
conducting analyses that could be impacted by the presence of related individuals as indicated
in the methods.
Population structure and dispersal barriers:
We assessed population structure among our samples using principal component
analysis (PCA). PCA results indicated that the one Indian sample was genetically distinct from
all of the other samples (Fig. 1C). Once removing two outlier samples, three distinct groups
became evident–Mongolia and Russia; Kyrgyzstan and captive; and Tajikistan, Afghanistan and
Pakistan (Fig. 1D).
(which was not certified by peer review) is the author/funder. All rights reserved. No reuse allowed without permission.
bioRxiv preprint doi: https://doi.org/10.1101/2023.12.14.571340; this version posted December 15, 2023. The copyright holder for this preprint
5
We also investigated population structure using Admixture30 to test models with one to
ten ancestral populations (K=1-10) over ten iterations. Admixture analyses can be sensitive to
differences in sample sizes among groups31, so the India sample was not included in this
analysis. At K=2, Mongolia and Russia separated clearly from all other samples, with the same
ancestry assignments supported in all ten iterations. At K=3, nine of the ten iterations supported
a clear separation of the samples into three groups–Mongolia and Russia; Tajikistan,
Afghanistan, and Pakistan; captives and Kyrgyzstan (Fig. 1B), recapitulating what we observe in
the PCA. These Admixture and PCA results are robust to the removal of captive samples from
the analyses (Supplementary Fig. 3).
We used EEMS (Estimated Effective Migration Surfaces)32 to assess migration patterns.
EEMS results were consistent with PCA and Admixture analyses and also indicated a
geographic barrier to migration from Kyrgyzstan to the northeast, around the Dzungarian Basin,
and to the south, around the Taklamakan Desert (Fig. 1E).
Taken together, our results suggest three genetically distinct groups within our samples
(excluding India). We see a primary genetic divide between the north (Mongolia and Russia)
and the south (all other samples) with a secondary divide within the southern group between
Kyrgyzstan and populations farther south (Afghanistan, Tajikistan, and Pakistan).
Assessment of gene flow:
Based on Admixture and PCA results, we quantified population structure between the
two groups identified in Admixture at K=2 and among the three groups identified at K=3 (Fig.
1B) by assessing shared versus private SNPs, FST, and rare allele sharing. Captive samples
and the Indian sample were excluded from these analyses. One sample from each related pair
was excluded from FST and rare allele sharing analyses, but not shared versus private SNP
assessments.
At K=2, each group had more shared SNPs (600,052 SNPs) than private SNPs (415,931
SNPs private to the north and 362,407 SNPs private to the south) (Supplementary Fig. 5A). At
K=3, we compared groups that we will refer to as “North” (consisting of Russia and Mongolia),
“Kyrgyzstan”, and “Far South” (consisting of Tajikistan, Afghanistan and Pakistan). The North
group was downsampled to eight individuals such that all three groups had a similar sample
size (Far South N=8, Kyrgyzstan N=7). We found that the Far South and North group had more
than twice as many private SNPs (234,825 and 224,417, respectively) than Kyrgyzstan
(109,016) (Supplementary Fig. 5B) and that Kyrgyzstan shared a similar number of SNPs with
both the North and the Far South (398,843 SNPs shared among all three groups, 64,319 shared
(which was not certified by peer review) is the author/funder. All rights reserved. No reuse allowed without permission.
bioRxiv preprint doi: https://doi.org/10.1101/2023.12.14.571340; this version posted December 15, 2023. The copyright holder for this preprint
6
only between Kyrgyzstan and North, 63,286 SNPs shared only between Kyrgyzstan and Far
South). It is worth noting that the Kyrgyz group represents the smallest geographic area and this
limitation in sampling could contribute to our observation of fewer private SNPs in this group.
Pairwise FST analyses indicated low levels of differentiation among the groups. Weir and
Cockerham’s pairwise FST was calculated after removing one individual for each first or second
degree related pair. At K=2, FST between the north and south was 0.091. At K=3, the pairwise
FST between the North and Far South was 0.123, between North and Kyrgyzstan was 0.108,
and between Kyrgyzstan and the Far South was 0.091.
Doubletons (SNPs with an alternate allele count of two), where each alternate allele
occurs in separate individuals, were identified. Doubleton sharing was then assessed among
the three groups–North, Kyrgyzstan, and Far South–after downsampling each group to six
individuals as in33. The amount of doubleton sharing between populations can offer a sense of
population connectivity as diverged populations have very little rare variant sharing34. Observed
doubleton sharing was compared to a null distribution representing panmixia created by
permuting the data 10,000 times. Doubleton sharing corroborated the pre-identified groups with
individuals sharing a significantly higher fraction of doubletons (0.40-0.72) with individuals within
the same group (p-value North – 0.0006, Kyrgyzstan – 0.008, Far South – 0.01) (Figure 1F).
However, we also found that each individual shares 11-32% of their doubletons with each other
group, with the Kyrgyzstan population showing the highest fraction of doubleton sharing with
outside groups – even sharing enough with the Far South to not be significantly different from
panmixia (p = 0.2). Both Kyrgyzstan and Far South also share enough rare alleles with the
North to only be marginally significantly different from panmixia (p=0.04 and p=0.03,
respectively). These results support the presence of genetic divergence among these three
groups, but also indicate a lack of any absolute barriers as there is some level of gene flow
among these groups.
(which was not certified by peer review) is the author/funder. All rights reserved. No reuse allowed without permission.
bioRxiv preprint doi: https://doi.org/10.1101/2023.12.14.571340; this version posted December 15, 2023. The copyright holder for this preprint
7
Figure 1. Snow leopard distribution and sample map, Admixture, principal components
analysis (PCA), estimated migration surfaces, and rare allele sharing. A) The IUCN snow
leopard distribution is shown and sample locations are indicated with different sized circles
indicating the number of samples from each location. The basemap indicates elevation and
country outlines are shown in grey. B) Admixture results for ten independent runs for K=2 and
K=3 distinct ancestry groups. The ancestry assignments shown for K=2 were supported by all
ten iterations and the ancestry assignments shown for K=3 were supported by nine of the ten
iterations. C) PCA of genetic variation of 37 snow leopards using 1,448,657 SNPs. D) PCA after
removing two outlier samples, India and sample U13 from Tajikistan. PCA axis labels include
the percent variation explained by PC1 and PC2. E) Estimate of migration surfaces based on
1,343,552 autosomal SNPs for 31 snow leopards. Log 10-transformed effective migration rates
are shown where green indicates areas with relatively higher migration and brown indicates
areas with relatively lower migration. Black circles represent sample locations as assigned to
their closest deme. Country outlines are shown in solid gray and landscape features are shown
(which was not certified by peer review) is the author/funder. All rights reserved. No reuse allowed without permission.
bioRxiv preprint doi: https://doi.org/10.1101/2023.12.14.571340; this version posted December 15, 2023. The copyright holder for this preprint
8
in dashed black lines and labeled (Dzungarian Basin (DB), Taklamakan Desert (TD), Gobi
Desert (GD)). F) Doubleton sharing between populations compared to null distributions under
panmixia. We identified all doubletons where each allele occurred in a different individual. For
each group, identified above each graph, we then calculated the fraction of doubletons
occurring in an individual of that group that were shared with individuals of each other group.
Null distributions were made by permuting the data 10,000 times. P-values for comparisons
between observed data and the null distribution using the Wilcoxon Rank Sum Test are shown.
The lower and upper edges of the boxes correspond to the first and third quartiles and the
whiskers extend to the lowest/highest value that is no further than 1.5*IQR (inter-quartile range)
from the box. Points falling further than 1.5*IQR from the box are plotted individually.
Heterozygosity and historic population size:
We used publicly available data to call SNPs in all big cat species using the Genome
Analysis Toolkit (GATK)35 and calculated heterozygosity using VCFtools36. Here, we defined big
cat species as species with an average adult body weight of 40kg or more, which includes all
Panthera species (lion (Panthera leo), tiger (Panthera tigris), leopard (Panthera pardus), jaguar
(Panthera onca), and snow leopard) as well as cheetah (Acinonyx jubatus) and puma (Puma
concolor) 37. We found snow leopards to have the lowest heterozygosity of any big cat species,
with heterozygosity for every snow leopard sample lower than that observed in any other big cat
(Fig. 2A). Notably, snow leopard heterozygosity was lower than that of cheetahs, which have
long been considered the archetype of low heterozygosity in big cats11,38. The relative values of
observed heterozygosity among all the other species for which we calculated heterozygosity
were consistent with previous work12,39–41. Within snow leopards, we found observed
heterozygosity to be consistently low across all sampled locations (Fig. 2B).
We used pairwise sequentially Markovian coalescent (PSMC)42 to reconstruct historic
effective population size using the highest coverage individual from each genetically distinct
group (North-28X, Kyrgyzstan/captive-28X, South-9X, India-12X). Reconstructions showed
consistent results across all populations sampled and across all bootstrap replicates (Fig. 2C).
All reconstructions show a consistently small effective population size over the last ~300,000
years with snow leopard effective population size never exceeding 14,000 individuals.
Reconstructions suggested a snow leopard effective population size of 8,000-6,000 individuals
from ~200,000-20,000 years ago and a decrease to an effective population size of ~2,500-4,000
individuals ~20,000 years ago, potentially coincident with the end of the last glacial maximum.
Effective population size is generally smaller than census size43 and PSMC historic effective
population size estimates can be impacted by numerous species-specific parameters (e.g.,
population structure, inbreeding, mutation rate, generation time)44,45 which have not been
(which was not certified by peer review) is the author/funder. All rights reserved. No reuse allowed without permission.
bioRxiv preprint doi: https://doi.org/10.1101/2023.12.14.571340; this version posted December 15, 2023. The copyright holder for this preprint
9
thoroughly characterized in snow leopards, and thus exact effective population size estimates
should be interpreted cautiously.
(which was not certified by peer review) is the author/funder. All rights reserved. No reuse allowed without permission.
bioRxiv preprint doi: https://doi.org/10.1101/2023.12.14.571340; this version posted December 15, 2023. The copyright holder for this preprint
10
Figure 2. Genome-wide heterozygosity and demographic history. A) Comparison of
genome-wide heterozygosity across all big cat species. Publicly available data was used to call
SNPs for every big cat species using GATK and observed heterozygosity was calculated using
VCFtools. In the case of leopard and tiger, SNPs were called separately for genetically distinct
groups. Snow leopard heterozygosity is calculated from SNPs called using the same in-house
pipeline that was used for SNP calling in all the other big cat species B) Genome-wide
heterozygosity of snow leopards separated by geographic location. Genome-wide
heterozygosity shown is calculated from SNPs called by Gencove. A direct comparison of
heterozygosity calculated from SNPs called in-house versus SNPs called by Gencove is shown
in Supplementary Fig. 6. The lower and upper edges of the boxes correspond to the first and
third quartiles and the whiskers extend to the lowest/highest value that is no further than
1.5*IQR (inter-quartile range) from the box. Points falling further than 1.5*IQR from the box are
plotted individually. C) Reconstruction of effective population sizes using PSMC using a
mutation rate of 0.7×10-8 per site per generation and a generation time of five years. Thirty
bootstraps are shown for each sample in thinner, fainter lines of the same color.
Discussion:
Heterozygosity and demographic history:
We used whole genome sequencing from 37 snow leopards to investigate genetic
diversity, demographic history, and population structure of the species. Our results show that
snow leopards have the lowest genetic diversity of any big cat species (Fig. 2A), lower than
previously appreciated12,46. Demographic history assessments indicated the exceptionally low
heterozygosity in snow leopards is likely due to a persistently small population size over the last
500,000 years (Fig. 2C). With a persistently small population size and low genetic diversity
throughout their evolutionary history, snow leopards serve as yet another example that high
genetic diversity is not a requirement for the long-term persistence of a species47–50. In light of
our results, very low population densities, as estimated in many contemporary landscapes (<0.5
animals/100 km2; e.g.,Suryawanshi et al.51), appears to be a familiar condition in snow leopard
evolutionary history.
Population structure and dispersal barriers:
PCA analyses show that India is genetically distinct from all other samples (Fig. 1C).
The uniqueness of this sample could suggest that this individual is our one representative of the
third phylogenetic group suggested by Janečka et al.27, but more samples from this area will be
necessary to resolve the unique evolutionary position of Indian snow leopards in future
analyses.
Among the other samples, we identify three genetically distinct groups. Structure and
PCA results identify the most pronounced divide among our samples to occur between the
(which was not certified by peer review) is the author/funder. All rights reserved. No reuse allowed without permission.
bioRxiv preprint doi: https://doi.org/10.1101/2023.12.14.571340; this version posted December 15, 2023. The copyright holder for this preprint
11
northern and southern part of the range around the Dzungarian Basin (Fig. 1B,D), consistent
with previous microsatellite analyses27 and resistant kernel models18. Our Structure and PCA
results also identify a secondary divide occurring south of Kyrgyzstan (Fig. 1B,D), around the
Taklamakan Desert, consistent with previous microsatellite analyses52. Both of these genetic
divides were also apparent in effective migration surface maps (Fig. 1E).
These results indicate that the Dzungarian Basin and Taklamakan Desert are effective
barriers to dispersal for snow leopards. However, low levels of differentiation – measured
through FST, shared versus private alleles (Supplementary Fig. 5), and shared rare allele (Fig.
1F) analyses – indicate that there is likely still some level of functional connectivity among these
three distinct groups despite intervening lowlands and large geographic distances between
them. Additional samples will be necessary to confidently estimate gene flow in future analyses.
Origin of captive samples:
Studbook-based pedigrees for the five captive individuals sequenced in this study show
that the origin of their wild ancestors is mostly unknown. The few ancestors of known origin are
documented as originating from the USSR, Kyrgyzstan, Kazakhstan, and the western
Himalayas (Supplementary Fig. 1). Admixture and PCA results both show a strong signal of
genetic relatedness between the five captive individuals and the current Kyrgyzstan population
(Fig. 1B,D). These results suggest that many of the wild ancestors listed as coming from the
USSR or unknown wild locations likely came from the Kyrgyzstan region.
Conservation implications:
With these data, we have begun to visualize the geographic distribution of genomic
diversity in snow leopards. Though this analysis does not contain samples from every part of the
snow leopard range (i.e., China and eastern Himalayas), it provides substantial insight into the
genetic divides across the portion of the range that we have sampled. We also provide evidence
that even the most expansive low-elevation areas are likely circumventable or traversable by
snow leopards, as our results suggest gene flow across all regions sampled. This indicates that
as human development continues and border fencing proliferates in this area17,53, it will be
important to maintain the likely narrow and dispersed corridors between distant patches of snow
leopard habitat to maintain existing levels of connectivity17.
Snow leopards live in arid, cold, low-productivity, high-elevation habitats where few
species can persist – an environment that has evidently only ever been able to support a limited
number of snow leopards. As a result, they have likely always had an effective population size
(which was not certified by peer review) is the author/funder. All rights reserved. No reuse allowed without permission.
bioRxiv preprint doi: https://doi.org/10.1101/2023.12.14.571340; this version posted December 15, 2023. The copyright holder for this preprint
12
much lower than other big cats (as suggested in Pečnerová et al.12 and Cho et al.14), and harbor
less genetic diversity than even the cheetah.
Thanks to their extreme environment, snow leopards have not yet been exposed to the
same level of acute Anthropogenic pressures as have big cats living in habitats more easily
accessible to humans. Yet, even having been spared the most intense human impacts, they
already have extremely low population size and genomic diversity. While these characteristics
have not hindered their long-term persistence thus far, this means that snow leopards do not
have the ability to rely on a large population size or standing genetic variation to help them
survive any forthcoming Anthropogenic challenges, as other big cats have done54,55.
Unfortunately, it is likely that the most intense Anthropogenic pressures may lie ahead for snow
leopards. In addition to their ongoing threats, Anthropogenic climate change threatens to shrink
snow leopard range through habitat change8 and intensify interspecific competition (e.g. Pal et
al.56, Lovari et al. 57); shifting grazing practices risk facilitating spillover of novel pathogens from
domestic animals into snow leopards and their prey58; and accelerating mining, energy, and
infrastructure development threaten to fragment and degrade previously remote snow leopard
habitats59,60. Our results reveal exceptionally low genetic diversity in the species, and make
clear that the persistence of snow leopards is likely tenuous. Protection of snow leopards and
their habitat, to the greatest degree possible, will be pivotal as this specialist, low-density
carnivore appears genetically and demographically ill-equipped to bounce back from
Anthropogenic perturbations61. More broadly, our findings underscore how the IUCN Red List
assessment criteria could benefit from the inclusion of genetic factors62 as our results suggest
that snow leopards may be more imperiled than their current IUCN Red List status would
indicate3,63.
Methods:
Sample Collection:
Through an international collaborative effort, a total of 37 snow leopard blood or tissue
samples were collected, composed of 33 wild caught samples from six countries (Afghanistan,
Kyrgyzstan, Tajikistan, Mongolia, and Russia), one currently captive but wild-born individual
from Pakistan, and four captive samples from mixed ancestry. Sample details can be found in
Supplementary Table 1. We were unable to include any samples from a large portion of the
snow leopard range in the southeast, but are hopeful that data from this area will be available to
include in future analyses. All samples were sequenced on an Illumina sequencing platform
using paired end 150 bp reads. Additionally, we collected all currently published whole genome
(which was not certified by peer review) is the author/funder. All rights reserved. No reuse allowed without permission.
bioRxiv preprint doi: https://doi.org/10.1101/2023.12.14.571340; this version posted December 15, 2023. The copyright holder for this preprint
13
sequencing data for snow leopards, which included data for two captive individuals (NCBI
accessions SRR1622751515 and SRR12437590), one wild individual from Mongolia (NCBI
accession SRR83637214), and one wild individual from India (NCBI BioProject PRJNA1051290).
In total, we gathered whole genome sequencing data for 41 snow leopards.
Note that there is whole genome sequencing data for an additional sample identified as
a snow leopard on Genbank (biosample SAMN17432540, SRA accession SRR13500277, used
in the following publications: Zhou et al.64,65) that we did not use in this study because we
concluded that this data was from a leopard (Panthera pardus), not a snow leopard (details of
the analyses done to identify this sample as a leopard are provided in Supplementary File 1).
Mapping and variant calling:
We used the snow leopard reference genome (NCBI accession PRJNA60293815) and
added the lion Y chromosome (from GCA_018350215.1) in order to have the Y-chromosome
represented in this female reference genome. Reads were mapped and variants called by
Gencove using BWA-MEM66 and the Genome Analysis Toolkit (GATK)67, respectively. The
depth and breadth of coverage for each sample were calculated from bam files using
SAMtools68 and are provided in Supplementary Table 1.
Variant filtering:
Variants were first filtered based on mappability across the genome. The reference
genome was indexed and mappability scores generated using genmapv1.3.069 with flags ‘-K 30’
and ‘-E 2’. The reference genome and mappability bed file were sorted using BEDTools70 and
SNPs falling in a region of the genome with a mappability score less than one were removed
using BEDTools intersect with the flags ‘-v’, ‘-header’, and ‘-sorted’ (leaving 63,199,070 sites).
We then used VCFtools36 to remove indels using the option ‘–remove-indels’ (leaving
51,199,725 SNPs). Individuals with less than 60% of the genome represented (samples U02,
U12, and U20) were removed using the VCFtools option ‘–remove-ind’. We then used
VCFtools to remove sites that no longer had any variation using flag ‘–non-ref-ac-any 1’ (leaving
2,379,069 SNPs), SNPs that did not fall on putative autosomes (leaving 2,213,174 SNPs), and
non-biallelic SNPs using th flags ‘–min-alleles 2 –max-alleles 2’ (leaving 2,146,294 SNPs). We
also split scaffold 22 at base pair 67,650,000 into chromosomes E1 and F1, respectively.
Next, we used GATK v4.1.4.1 to add the following annotations to our filtered vcf file using
VariantAnnotator: QD (QualByDepth – variant confidence normalized by unfiltered depth of
variant samples), FS (FisherStrand – strand bias estimated using Fisher’s exact test), MQ
(which was not certified by peer review) is the author/funder. All rights reserved. No reuse allowed without permission.
bioRxiv preprint doi: https://doi.org/10.1101/2023.12.14.571340; this version posted December 15, 2023. The copyright holder for this preprint
14
(RMSMappingQuality – root mean square of the mapping quality of reads across all
samples), MQRankSum (MappingQualityRankSumTest – rank sum test for mapping
qualities of reference versus alternate reads), and ReadPosRankSum
(ReadPosRankSumTest – rank sum test for relative positioning of reference versus alternate
alleles within reads). After adding these annotations, we used GATK VariantFiltration to
filter SNPs using the following flag: ‘–filter-expression “QD < 2.0 || FS > 60.0 || MQ < 40.0 ||
MQRankSum < -12.5 || ReadPosRankSum < -8.0″’. We then used VCFtools to remove
SNPs that did not pass the GATK VariantFiltration step using option ‘–remove-filtered-all’
(leaving 2,065,453 SNPs). Lastly, we removed SNPs missing data in more than 10% of the
individuals using flag ‘–max-missing 0.9’ (leaving a final SNP set of 1,591,978). Singletons and
private doubletons were calculated using the VCFtools flag ‘–singletons’ and one of the captive
samples (10x_SDzoo) was removed due to an excess of singletons (Supplementary Table 1)
which we suspected to be due to the 10X library prep method unique to this sample.
Relatedness assessment:
Relatedness among samples was estimated using SNPrelate71 in R72. We divided the
samples into three genetically distinct groups based on preliminary PCA and Admixture
analyses–Mongolia and Russia, Kyrgyzstan and captive, and all samples South of Kyrgyzstan.
Preliminary PCA and Admixture analyses were done using the same methods described below
but in this case included samples that were later removed due to relatedness. Each group was
run through SNPrelate separately. We used the function snpgdsBED2GDS to convert bed files
to gds files. We used snpgdsLDpruning to prune the input data using the following flags –
‘method=”corr”, slide.max.n=50, ld.threshold=0.3, maf = 0.05, autosome.only = F’. We then
calculated kinship coefficients using the function snpgdsIBDMLE which calculates IBD
coefficients for individual pairs using Maximum Likelihood Estimation. A kinship coefficient of 0.5
indicates a duplicate sample, 0.25 indicates a first-order related pair (parent-offspring or full
sibling), and 0.125 indicates a second-order related pair (half-siblings or grandparentgrandchild). All sample pairs with non-zero kinship coefficients are listed in Supplementary
Table 2. We identified one sample pair with a kinship coefficient greater than that expected from
first-order relatives–U01 and U09 (kinship coefficient of 0.335), and we identified three sample
pairs with kinship coefficients consistent with second-order related pairs–SL_KGZ_F1 and
SL_KGZ_F4 (kinship coefficient of 0.148), AF_SL_07 and AF_SL_06 (kinship coefficient of
0.135), and U14 and U08 (kinship coefficient of 0.124). The sample with lower sequencing
(which was not certified by peer review) is the author/funder. All rights reserved. No reuse allowed without permission.
bioRxiv preprint doi: https://doi.org/10.1101/2023.12.14.571340; this version posted December 15, 2023. The copyright holder for this preprint
15
coverage from each of these pairs (U01, SL_KGZ_F4, AF_SL_06, and U08) was removed from
indicated analyses.
Pedigree-based measures of relatedness in captive-bred samples:
Using snow leopard studbooks73,74, we compiled information on all of the ancestors of
the five captive-born individuals included in this study. The wild origin of their ancestors is
mostly unknown; however, each sample has a few ancestors for which there is a wild origin
location listed (Supplementary Fig. 1), these origin locations include wild ancestors listed as
coming from the USSR (between 1964-1974), Kazakhstan (in 1972), Kyrgyzstan (between
1974-1980), Prezewalsk (between 1974-1975, which we believe to refer to Karakol, Kyrgyzstan
which was previously named Prezewalsk), and Aksai (a contested region between China and
India in the Western Himalayas, in 1979).
Pedigrees for each individual were constructed (Supplementary Fig. 1) and kinship was
estimated (Supplementary Table 3) among these individuals using the kinship2 package75 in R.
Kinship calculated from pedigrees was compared to kinship calculated from SNPrelate using
Pearson correlation coefficient calculated using the ggpubr76 package in R and plotted using
ggplot277. We found kinship calculated from pedigrees to be highly correlated with kinship
calculated from SNPrelate (R = 0.91, p = 0.00023, Supplementary Fig. 2).
Principal component analysis:
Principal component analyses (PCAs) were conducted on the dataset after filtering for
first and second degree relatives (N=33, 1,448,657 SNPs) using PLINK278. First, bed files were
generated from vcf files using the flags ‘–make-bed’ and ‘–allow-extra-chr’ and allele
frequencies were computed using the flags ‘–freq’ and ‘–allow-extra-chr’. Then, the principal
component analysis was run by inputting the resultant bed file with flag ‘–bfile’ and frequency
file with flag ‘–read-freq’ and using the flags ‘–allow-extra-chr’ and ‘–pca 38’. PCA output was
visualized using ggplot2 in R (Fig. 1C,D).
Admixture analysis:
Admixture analyses were also conducted on the dataset after filtering for first and
second degree relatives. Since these analyses can be sensitive to uneven sample size, the
Indian sample was excluded from this analysis (N=32)31,79. We used VCFtools to convert vcf
files into PLINK format files. We then used PLINK1.9 to recode alleles numerically using the
flags ‘–allow-extra-chr –recode12’. We then ran Admixture30 for K1-10 ten times using the flag
(which was not certified by peer review) is the author/funder. All rights reserved. No reuse allowed without permission.
bioRxiv preprint doi: https://doi.org/10.1101/2023.12.14.571340; this version posted December 15, 2023. The copyright holder for this preprint
16
‘-s time’ so that a different random seed was used for each run based on the time. All runs were
then visualized using Clumpak80 (Fig. 1B).
PCA and Admixture analyses were also conducted on the dataset after the removal of
captive samples in order to assess the impact of captive samples on population structure results
(Supplementary Fig. 3).
Estimate of Effective Migration Surfaces (EEMS):
We used a matrix of average pairwise genetic dissimilarities as input into EEMS32 to
estimate effective migration and diversity surfaces across the snow leopard range. To make this
input file from the filtered vcf file, we used VCFtools to remove all captive-bred samples, the one
Mongolia sample (SRR836372) lacking location data, and one individual per first or second
degree related pair (U01, U08, AF_SL_06, SL_KGZ_F4) (N=27). We then removed sites for
which there was no longer any variation present in the remaining samples and were left with
1,343,552 SNPs as input into EEMS. We then used PLINK1.9 to create a bed file from the vcf
using the ‘–make-bed’ flag. Lastly, we used bed2diffs in EEMS to generate the pairwise
dissimilarity matrix from the bed file. The habitat outline used was selected to include the entire
extant range of snow leopards as shown in Fig. 1A and was drawn manually using arcgis online.
We ran runeems_snps with 8 million MCMC iterations, 1 million burn-in iterations, and 9999
iterations thinned between writing steps. We used grids of 200, 400 and 600 demes and
averaged the results across three independent realizations at each deme size. Through
numerous initial trial run iterations, we adjusted the proposed variance of parameters such that
the accepted proportions of proposed variances all fell between 10-40%. Results of all nine
independent runs were visualized together in R using the package rEEMSplots32 (Fig. 1E).
We also ran EEMS on the microsatellite dataset from27 which is composed of 32 variable
microsatellite loci genotyped for 70 individuals. We analyzed this dataset using runeems_sats
using the same parameters as above – 8 million MCMC iterations, 1 million burn-in iterations,
9999 iterations thinned between writing steps, and grid sizes of 200, 400, and 600 demes. Each
deme size was run three independent times and results were averaged across all nine
realizations (Supplementary Fig. 4).
Quantifying population structure:
We further characterized population divides identified in Admixture and PCA analyses by
calculating the number of shared versus private SNPs among groups, pairwise FST, and the rate
of rare variant sharing among groups. Captive samples were excluded from these analyses as
(which was not certified by peer review) is the author/funder. All rights reserved. No reuse allowed without permission.
bioRxiv preprint doi: https://doi.org/10.1101/2023.12.14.571340; this version posted December 15, 2023. The copyright holder for this preprint
17
was the sample from India as the PCA indicates that this sample is genetically distinct from all
groups.
We did not filter for relatedness when calculating shared versus private SNPs among the
groups; however, we did downsample the Northern group at K=3 such that all groups had
between 7-8 individuals. We used BCFtools81 to create and index vcf files with only the
individuals from each group using the view and index function, respectively. We then used
BCFtools isec to calculate how many SNPs were shared and private among the groups.
Before calculating FST, we removed one individual for each first or second degree related
pair. We first used PLINK1.9 to create transposed PLINK files using the flags ‘–allow-extra-chr –
-double-id –recode –transpose’. We then used PLINK2 to calculate FST among groups using the
Weir and Cockerham estimator82 using the flags ‘–allow-extra-chr –fst CATPHENO
method=wc’.
To assess doubleton sharing among groups, we excluded one individual for each related
pair and downsampled all groups so that each had an equal number of individuals (N=6). We
then used VCFtools to retain only SNPs with a minor allele count of two (doubletons) using the
flags ‘–mac 2 –max-mac 2’. We removed any SNPs where the doubletons were present in the
same individual and identified allele sharing among samples using the flag ‘–genome full’ in
PLINK. A null distribution under panmixia was created by permuting the data 10,000 times.
Fractions of observed doubleton sharing were compared to the null distribution using the
Wilcoxon Rank Sum Test in R.
PSMC:
We used PSMC42 to estimate snow leopard population size back in time using the
highest coverage sample for each population cluster. We generated diploid consensus
sequences for each individual as recommended by PSMC. Starting with bam files filtered to
include only putative autosomes, we used SAMtools mpileup and BCFtools call to generate a
vcf and then used vcfutils.pl vcf2fq to generate diploid fastq files. We then ran PSMC with the
default settings. We also performed 30 rounds of bootstrapping using random sampling with
replacement for each sample. For plotting, we used a mutation rate of 0.7×10-8 per site per
generation as suggested for snow leopards by Cho et al.14 and a generation time of five years.
Heterozygosity:
Observed homozygosity was calculated for every individual from the vcf file using
VCFtools36 with the flag ‘–het’. In R, we calculated the number of heterozygous sites by
(which was not certified by peer review) is the author/funder. All rights reserved. No reuse allowed without permission.
bioRxiv preprint doi: https://doi.org/10.1101/2023.12.14.571340; this version posted December 15, 2023. The copyright holder for this preprint
18
subtracting the number of observed homozygous sites column ((O)HOM) from the total number
of sites column (NSITES). We then calculated heterozygosity by dividing the number of
heterozygous sites by the length of the genome consisting of putative autosomes with a
mappability score of one (1,818,166,894). Heterozygosity was calculated for all individuals,
regardless of relatedness (N=37). We used ggplot2 in R to create box plots of heterozygosity
results. All snow leopard samples had a heterozygosity between 0.000039 – 0.000168 (Fig. 2B).
Heterozygosity across big cats:
Heterozygosity was calculated in all big cat species using publicly available data in order
to see how snow leopard heterozygosity levels compare to other big cat species. We included
all other species in the genus Panthera (leopard, lion, tiger, jaguar) as well as cheetah and
mountain lion. Accession numbers of publicly available whole genome sequencing data and
reference genomes used in this analysis are listed in Supplementary Table 4 and 5,
respectively. We also recalculated heterozygosity in all snow leopard samples using the same
in-house pipeline to ensure that heterozygosity calculated using SNPs called with the Gencove
pipeline were comparable to heterozygosity calculated using SNPs called with our own pipeline.
All fastq data was mapped to the corresponding reference genome using BWA-MEM66.
Resulting bam files were sorted and indexed using SAMtools68. Depth of coverage and breadth
of coverage was calculated for each sample using SAMtools. We added read groups and
marked duplicates using picard83. We then used GATK35 HaplotypeCaller to generate a gvcf file
for each sample and GATK CombineGVCFs to combine the gvcf files for every species (or
subgroup in the case of tigers and leopards). Lastly, we used GATK GenotypeGVCFs to create
a final vcf file for every group.
In order to limit the need for excessive computing power, some samples with over 30X
coverage were downsampled using SAMtools view as indicated in Supplementary Table 4. The
leopard samples required a larger amount of computing power than the other species to call
haplotypes, so these samples were all split into one bam file per contig using BAMtools split and
run through GATK HaplotypeCaller and CombineGVCFs in parallel. We also found it necessary
to split the jaguar and snow leopard data into intervals to combine gvcf files using GATK
GenomicsDBImport followed by CombineGVCFs. In both cases, resultant vcf files, one per
contig or interval, were then concatenated using BCFtools concat.
Each reference genome was filtered for mappability using genmapv1.3.0 to first index
and then calculate mappability using flags ‘-K 30’ and ‘-E 2’. We then filtered each vcf to only
include nucleotides with a mappability score of one. To do this, vcf files and mappability bed
(which was not certified by peer review) is the author/funder. All rights reserved. No reuse allowed without permission.
bioRxiv preprint doi: https://doi.org/10.1101/2023.12.14.571340; this version posted December 15, 2023. The copyright holder for this preprint
19
files were both sorted using BEDTools and only SNPs falling in regions with a mappability score
of one were retained in each vcf using BEDTools intersect. Each vcf was then filtered using
GATK VariantFiltration with the following flag: ‘–filter-expression “QD < 2.0 || FS > 60.0 ||
MQ < 40.0 || MQRankSum < -12.5 || ReadPosRankSum < -8.0″’. Then, vcf files were filtered
to remove indels and non-bialellic SNPs using VCFtools flags ‘–remove-indels –min-alleles 2 —
max-alleles 2’.
The mappable length of each genome was calculated using mappability bed files to
calculate the number of nucleotides with a mappability score of one. Using the filtered vcf for
each species or group, observed heterozygosity was then calculated for each sample and
plotted in the same way described above.
Snow leopard heterozygosity calculated from SNPs called using our in-house pipeline
was compared to heterozygosity calculated from SNPs called by Gencove using Pearson
correlation coefficient calculated using the ggpubr76 package in R (Supplementary Fig. 6). We
found heterozygosity calculated from the two different SNP calling pipelines to be extremely
correlated (R = 0.98, p=2.2E-16); however, all heterozygosity estimates calculated from our inhouse SNP calls were slightly higher (average of ~0.000041) than that estimated from Gencove
SNP calls.
Data Availability:
Data associated with this study has been deposited into bioproject PRJNA1048427 and will be
released upon publication.
Code Availability:
The code used for analyses in this project is available on the project’s github:
https://github.com/ksolari/SL_WGS
Acknowledgements:
We would like to thank those who helped us in acquiring the captive samples used in this study,
including Karen Ingerman and Susie Bartlett from the Bronx Zoo. We would like to thank
Haiqing Xu for his help in conducting simulations to produce the null distribution for the shared
doubleton analysis.